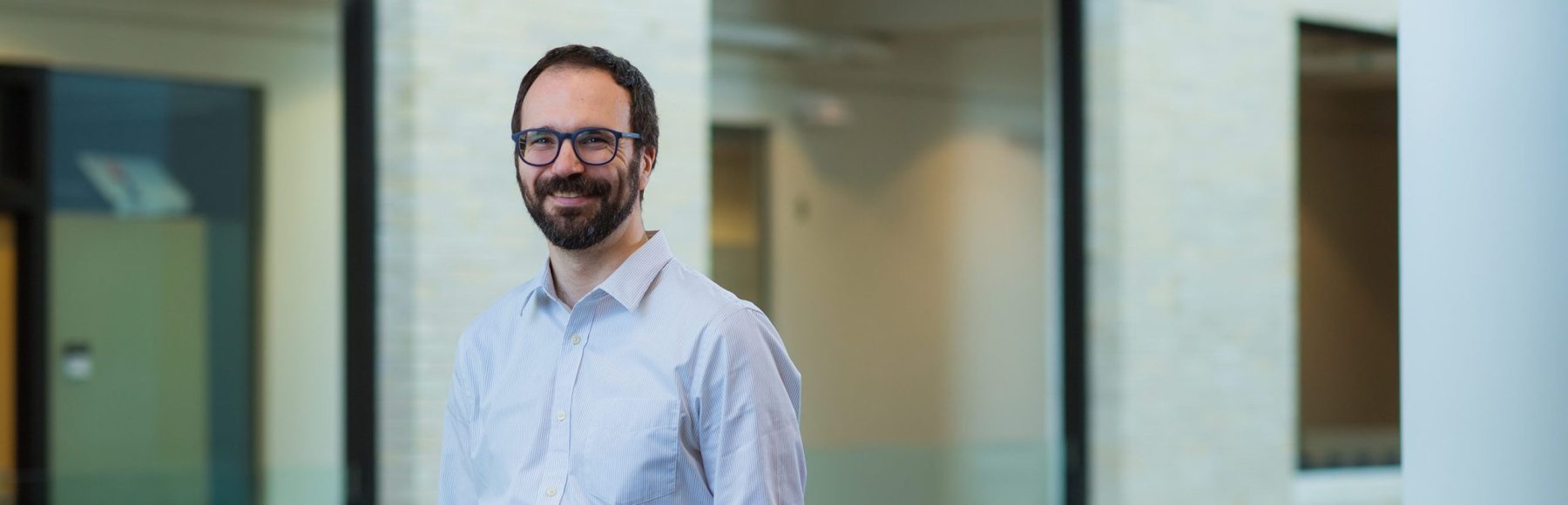
Rafael Gómez-Bombarelli
Primary Impact, Materials, Research Type
Contact Info
Research
Professor Rafael Gómez-Bombarelli’s research trajectory has evolved from doctoral work in experimental physical organic chemistry to computer-driven design of materials. By fusing physics-based atomistic simulation with machine learning models, he aims to accelerate the discovery cycle of novel practical materials. Professor Gómez-Bombarelli’s research interests are in understanding how composition, structure, and reactivity at the atomic scale relate to the performance of materials. By harnessing these design rules, his research group works on the discovery of materials for energy, sustainability, and health care; thermo- and electro-catalysts to organic and inorganic battery materials; sustainable polymers; and photoswitchable drugs.
Biography
Professor Gómez-Bombarelli received his BS, MS, and PhD in chemistry from the University of Salamanca in Spain, followed by postdoctoral work at Heriot-Watt University in Scotland. As a postdoc at the Aspuru-Guzik lab at Harvard University he worked on high-throughput virtual screening for organic light-emitting diode (OLED) and battery electrolytes. He entered industry in 2016 as a senior researcher at Japanese technology company Kyulux, applying Harvard-licensed technology to build commercial OLED products. He joined the DMSE faculty in 2018.
Professor Gómez-Bombarelli’s work has been featured in publications such as MIT Technology Review and the Wall Street Journal. He is co-founder of Calculario, a materials discovery company that uses quantum chemistry and machine learning to target advanced materials in a range of high-value markets.
Key Publications
A priori control of zeolite phase competition and intergrowth with high-throughput simulations
Discovered a better way of custom-designing zeolites, porous minerals that can trap unwanted molecules from gases or liquids.
Excited state non-adiabatic dynamics of large photoswitchable molecules using a chemically transferable machine learning potential
We developed a machine learning approach that accelerates the process of identifying photoswitches, molecules that activate, or “turn on,” when exposed to light.