Computation and Design
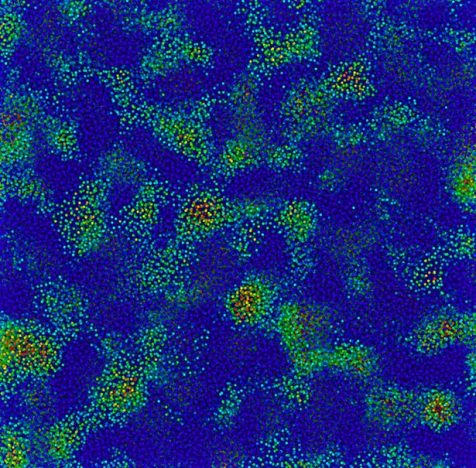
Speeding Up Materials Discovery
In materials science and engineering, computation and design involve the use of computational tools and methods to design new materials more quickly and efficiently. Researchers use computers to simulate material properties such as strength and electrical and thermal conductivity to understand how materials perform under different conditions and can inform the design of new materials with specific properties.
Computational methods and tools such as computer-aided design are used to create virtual models of new materials and algorithms to find the best combinations of material properties for an application. Machine learning and artificial intelligence techniques can also be incorporated to accelerate new materials discovery.
Computation and Design at DMSE
Materials scientists and engineers at DMSE are using mathematical models and simulations to understand how materials’ structure and composition affect their properties—how they conduct electricity, interact with light, and behave under extreme conditions such as high temperatures. They use this knowledge to design and create new materials with improved properties. DMSE researchers are also developing algorithms that can analyze vast amounts of data to identify new materials for various applications, ranging from electronics to energy storage and beyond.
Related Materials
Related Faculty and Researchers
Key Publications
Low-hysteresis shape-memory ceramics designed by multimode modeling
Created a category of shape-memory materials from ceramics that can operate at higher temperatures without sustaining much damage.
Excited state non-adiabatic dynamics of large photoswitchable molecules using a chemically transferable machine learning potential
We developed a machine learning approach that accelerates the process of identifying photoswitches, molecules that activate, or “turn on,” when exposed to light.