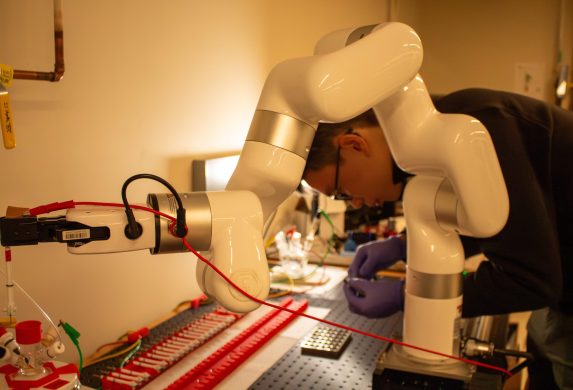
Almost any scientist will be grateful for an assistant in the lab—to save time and effort. Someone to fix equipment issues, figure out the next batch of experimental parameters to test, or take care of the grunt work involved in running experiments. After all, laboratory-based research is time-intensive and tedious.
Zhichu Ren, a PhD student in MIT’s Department of Materials Science and Engineering (DMSE), has developed a lab assistant that doesn’t mind the work or long hours: the Copilot for Real-World Experimental Scientist (CRESt). Powered by various types of artificial intelligence (AI), CRESt suggests experiments to try and guides researchers to the next steps in a process workflow. The assistant can retrieve and analyze data, switch equipment on and off, and drive robotic arms to mix liquids, for example, or prepare materials for experimentation or analysis.
The voice-based robotic system can “decrease soul-crushing benchwork and help experimentalists put more time into thinking,” said Ren’s advisor, Ju Li, the Battelle Energy Alliance Professor of Nuclear Science and Engineering and Materials Science and Engineering.
In a Nature Reviews Materials paper, Ren and Zhen Zhang, also a PhD student in DMSE, outline the hurdles that need to be overcome before such assisted experiments can take full flight. Li is the principal author of the study; other authors are Zekun Ren and Professor Tonio Buonassisi from MIT’s Department of Mechanical Engineering.
Saves time, eliminates tedious work
In any underexplored domain of science, striking gold is often a result of hundreds (if not thousands) of time-consuming experiments. For example, a recent project in Li’s lab involved converting carbon dioxide into formate, a material that can be used to power a fuel cell and generate electricity. The team built its own fuel cell that needed a special multi-material catalyst to speed up interactions and convert fuel into electricity.
Figuring out the precise composition of metals that could optimize the conversion process requires systematic evaluation of many permutations and combinations. Scientists must figure out whether they should explore untried combinations or fine-tune known ones that have delivered the best results.
Patiently chipping away at materials possibilities is a tried-and-tested approach, but when it comes to pressing problems such as climate change, scientists don’t always have the luxury of time, Li pointed out. In the carbon-to-formate example, scientists can save hours of research time if an assistant can figure out which metal combinations for a fuel cell catalyst to try next.
This is where the active learning component of CRESt kicks in. Active learning is a specialized form of machine learning. It’s like a student who learns more effectively by asking questions rather than passively listening. In this approach, the machine learning model selectively suggests new experiments to get information on results it finds confusing or uncertain.
As an example, the active learning component of CRESt uses a trained algorithm to select the next set of experimental parameters to evaluate in the renewable energy projects conducted in Li’s lab. For this work, active learning can suggest not just the composition of materials to use but also processing conditions such as temperature and how long a metal should undergo heat treatment. Essentially, the program learns from detailed information—materials used and processing conditions—from previous experiments to recommend a path forward. Such recommendations optimize effort.
Previously, the lab members had been choosing compositions themselves. “We had to figure out what to mix and then choose the processing parameters, but by using CRESt, we can do the least number of tests possible to achieve optimal results,” Li said.
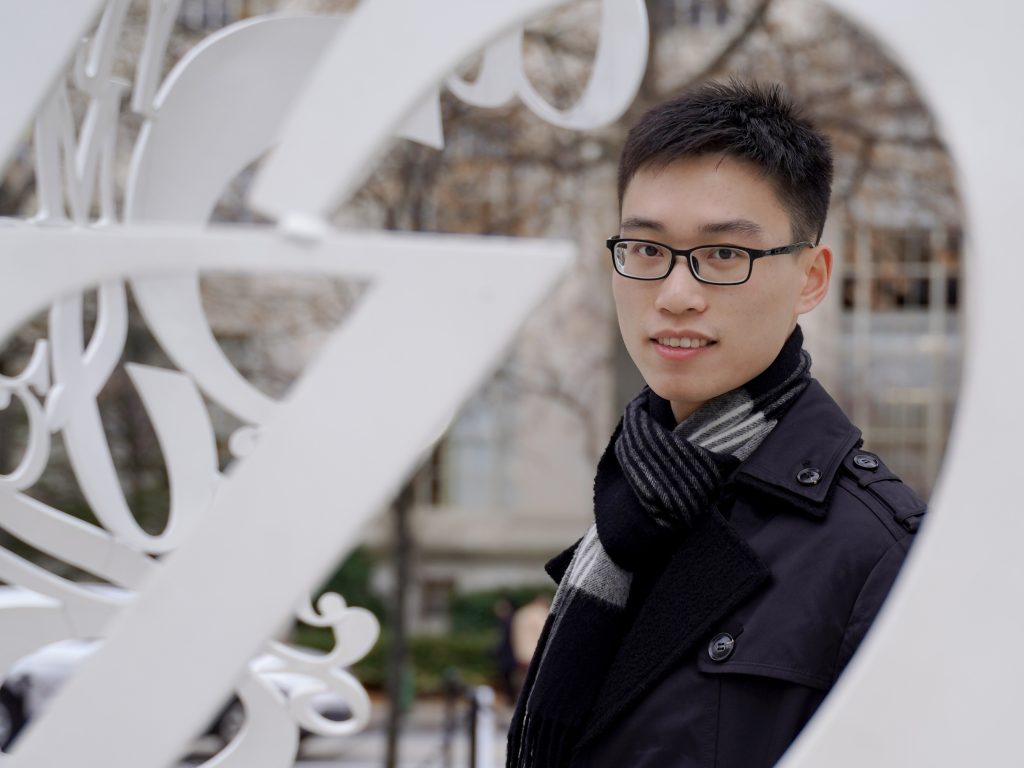
CRESt can also lessen the load of tedious work. The lab researcher need not be present late in the night to switch off the gas valve; CRESt can take care of it by sending a command to a digital valve wirelessly.
The assistant does so by incorporating generative AI, specifically the popular chatbot ChatGPT, allowing researchers to control experiments using voice commands. Here’s how it works: CRESt converts a researcher’s speech to text and taps ChatGPT’s recent calling function feature, which integrates the programming code that relays the command. The system then generates text responses that are translated into back into speech for the researcher. All this happens in seconds—a conversation between human and machine. In a video demonstration of CRESt, Ren instructs the system to mix liquid precursors, turn on a laser cutter and a pump, and switch the gas on.
Ren worked closely with active learning expert Yunsheng Tian from MIT’s Department of Electrical Engineering and Computer Science on applying mathematical methods in conjunction with active learning to tackle materials questions. In this ChemRxiv working paper from November, Ren and co-authors Zhang, Tian, and Li discuss in detail how CRESt works with ChatGPT to enable researcher interaction with the platform.
Even more recently, the group wrote a paper on fuel cell research conducted with CRESt that was presented at NeurIPS 2023, a computer science conference. The paper was featured as part of the “Adaptive Experimental Design and Active Learning in the Real World” workshop and earned the best paper award in December.
The future of experimentation
While CRESt is optimized for materials science experiments, the broader concept of autonomous labs can revolutionize experimental workflows in diverse scientific fields. Moreover, developing similar lab assistants would help researchers rethink experimental design, said Aldair Gongora, a staff scientist at Lawrence Livermore National Laboratory, who works on self-driving labs.
Until recently, scientists have mostly harbored a human-centric perspective to experiments—from the size of the samples needed for human hands to work with to the number of analyses scientists can reasonably conduct in a given amount of time.
With AI assisting them in their experimentation, researchers will need to rethink how much energy, time, and money are needed to conduct a meaningful science experiment. On the flip side are questions about how to scale autonomous labs and workflows and apply them to industrial processes, urgently needed for the ongoing transition away from the fossil-fuel-powered economy.
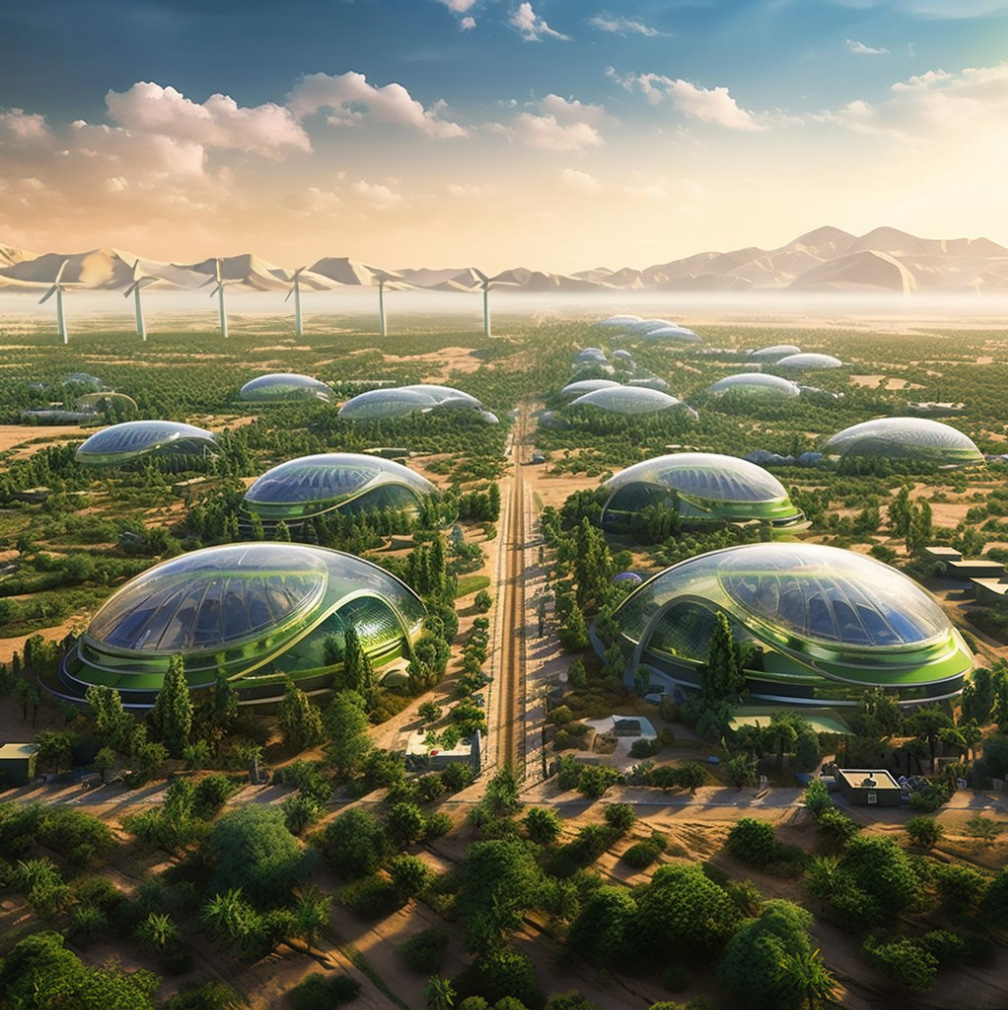
Matters of large-scale applicability of such research excite Ren and Li. Ren is enthusiastic about the possibility of the “mass production of science” to combat pressing problems like climate change.
AI is going to be a game-changer, Li said. “With large language models and active learning and computer vision and robotics, things seem to be at a turning point in lab-based research.”
The need to establish new standards is crucial for every kind of autonomous experiment. This includes everything from making sure samples can be transferred easily between different labs to conducting fair testing and reviewing procedures, Li said. Building this framework will allow cloud-based labs to share data and work on solutions together, accelerating the pace of combating climate change.
And the potential of cloud-based technologies for data sharing is huge, Gongora said. “It will give us a collective sense of how we can approach problems as a community.”
For the immediate future, though, humans are good at diagnosing and turning experimental failures around; AI is less so. Later, these technologies might be able to self-diagnose and self-correct mistakes, but for now, they need heavy human intervention. “Today’s AI is like a kid and still stumbles, so we have to have good parenting skills,” Li said.
But once it reaches maturity, expect AI to tackle big challenges quickly. With autonomous experiments forming the basis for self-driving and collaborative labs, researchers across disciplines can pool resources to address big challenges.
“The future is about having automated experimentation, machine learning, and the human in the autonomous experimentation loop,” Gongora said. The kind of autonomous experiments that the MIT team is working on is what’s needed to shape the labs of tomorrow.