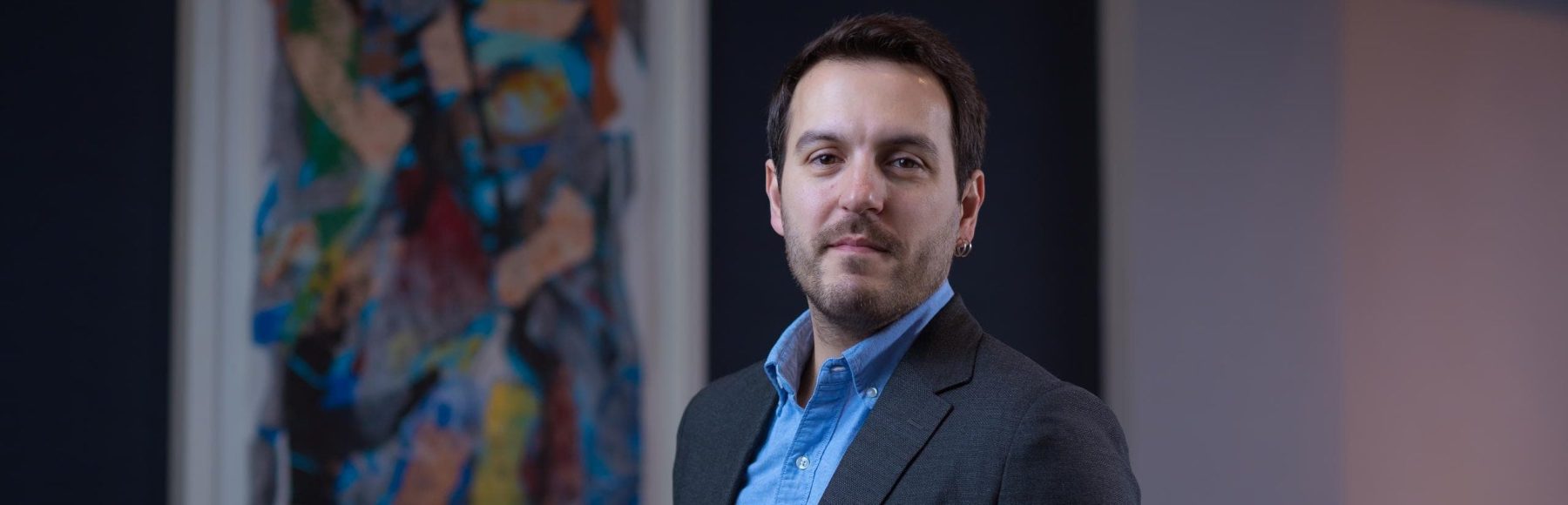
Rodrigo Freitas
Primary Impact, Materials, Research Type
Contact Info
Research
Professor Rodrigo Freitas’ research focus is on elucidating the fundamental mechanisms of microstructural evolution in structural materials such as metals but also in other systems of relevance in materials science broadly construed. His research group employs a range of computational and data science techniques with the goal of bridging the gap between the all-atom information gathered from simulations and the mesoscale description of microstructural elements employed in materials science.
Biography
Professor Freitas received a BS and an MS in physics from the University of Campinas in Brazil, and master’s and doctoral degrees in materials science and engineering from the University of California, Berkeley. During his PhD he was also a Livermore Graduate Scholar in the materials science division of the Lawrence Livermore National Laboratory. As a graduate student, Professor Freitas investigated the thermodynamics, kinetics, and mechanics of extended defects in metals (such as grain boundaries and dislocations) using atomistic simulation—methods that explicitly consider the behavior of each atom. As a postdoctoral researcher at Stanford University, he worked to leverage machine learning tools to perform physics-based modeling of materials kinetics.
Key Publications
Uncovering the effects of interface-induced ordering of liquid on crystal growth using machine learning
Employed machine learning methods and simulations to understand the role of the liquid in the solidification process.